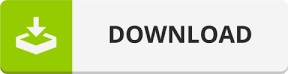
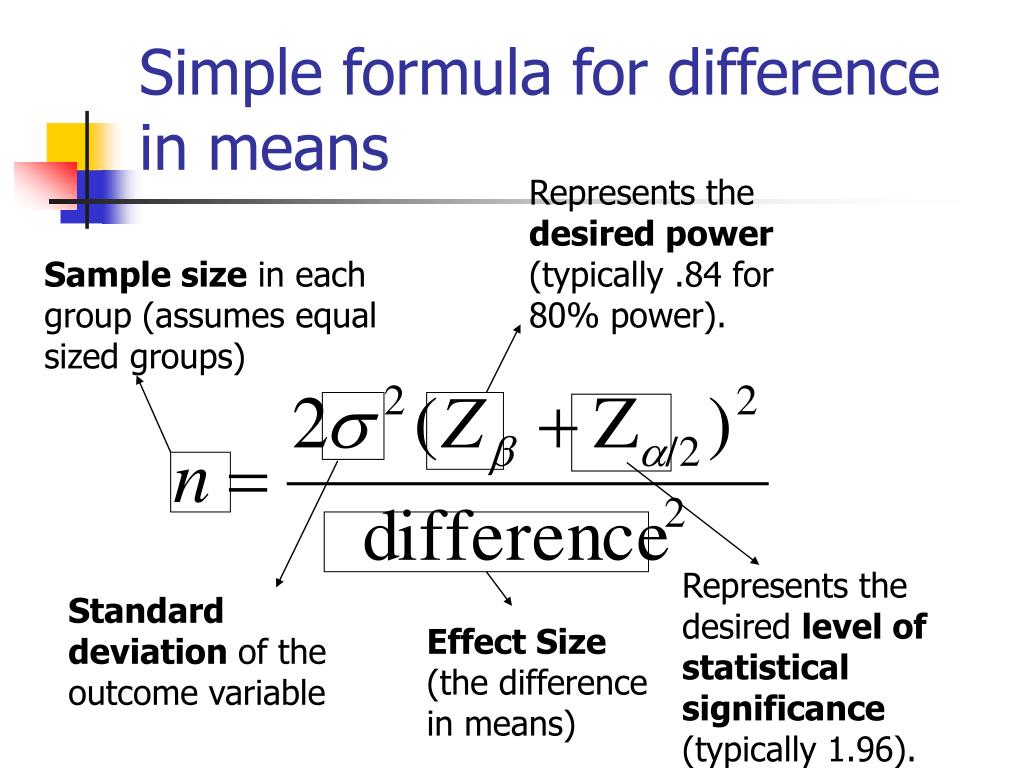
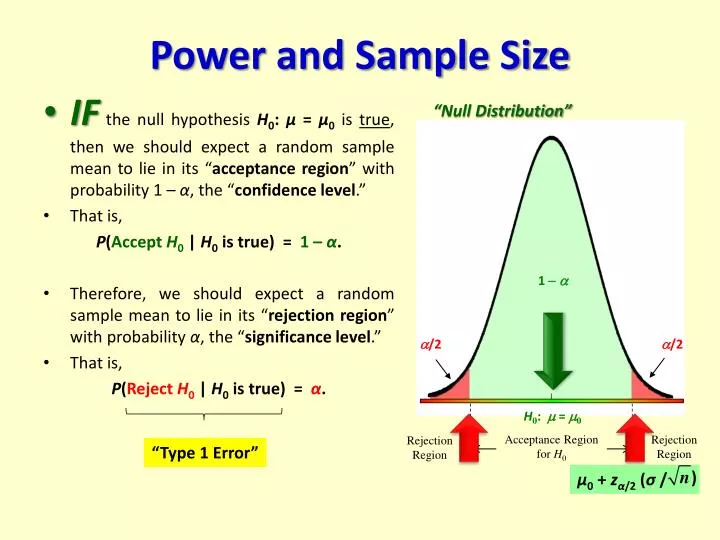
Thus, our aim is to provide tools and resources for researchers to use and explore simulation-based procedures, empowering them to find solutions for their own specific use cases. Consequently, all scenarios introduced in this tutorial assume that an optimal model has already been selected. This paper is designed to meet the needs of researchers who have some experience with mixed-effects modeling, and thus does not discuss topics such as model selection or optimal random-effects structure (Barr, Levy, Scheepers, & Tily, 2013 Bates, Kliegl, Vasishth, & Baayen, 2015a).
#Sample size power analysis how to
In this tutorial, we will consider different scenarios for a simulation-based power analysis and provide examples on how to perform such an analysis. However, accounting for statistical power while planning experimental designs is important for the reliability and replicability of empirical findings and a critical step for the successful preregistration of studies. Baayen et al., 2008 Bates, Mächler, Bolker, & Walker, 2014 DeBruine & Barr, 2021 Goldstein, 2007 Harrison et al., 2018), but far less guidance is available for experimental psychologists who want to estimate power for (G)LMMs (Brysbaert & Stevens, 2018 Westfall, Kenny, & Judd, 2014).
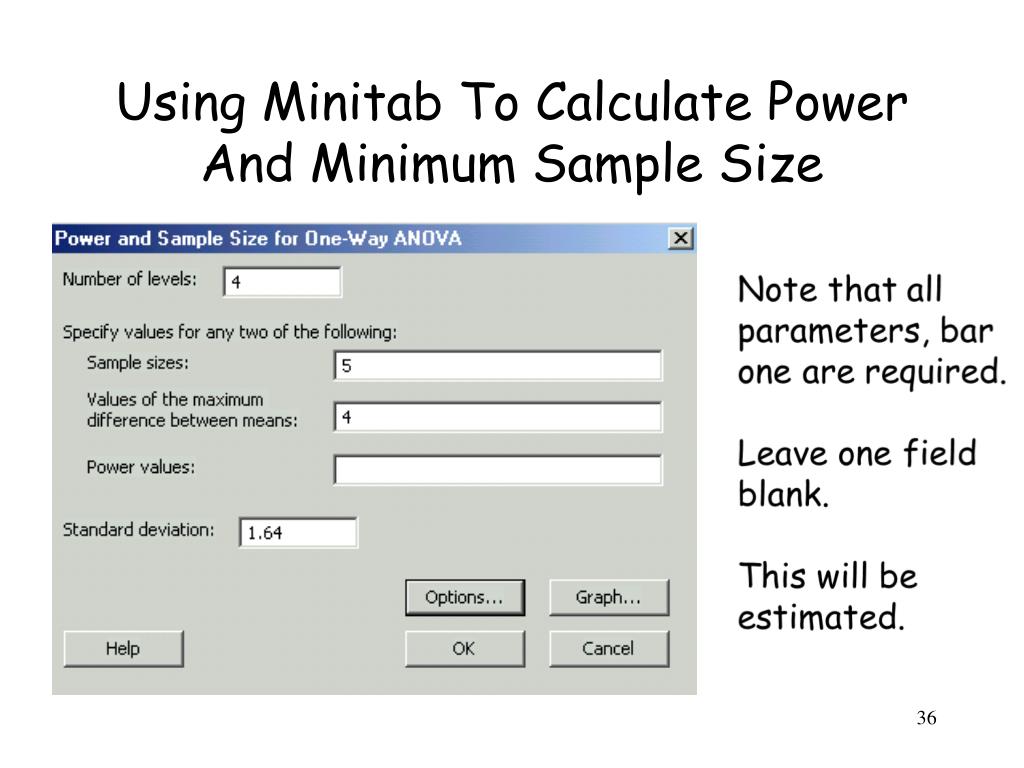
There are plenty of excellent resources aimed at using (G)LMMs (e.g. Moreover, (G)LMMs offer flexibility in dealing with missing data and unbalanced designs, and allow for a unified treatment of continuous and categorical responses (Baayen et al., 2008). Linear mixed-effects models (LMMs), as well as generalized linear mixed models (GLMMs), are a popular and powerful choice in cognitive research, as they allow between-subject and between-item variance to be estimated simultaneously (for a discussion see Baayen, Davidson, & Bates, 2008 Kliegl, Wei, Dambacher, Yan, & Zhou, 2011). The present work therefore helps researchers to navigate sound research design when using mixed-effects models, by summarizing resources, collating available knowledge, providing solutions and tools, and applying them to real-world problems in sample sizing planning when sophisticated analysis procedures like mixed-effects models are outlined as inferential procedures.
#Sample size power analysis code
Our examples cover both linear and generalized linear models and we provide code and resources for performing simulation-based power analyses on openly accessible data sets. published) data to determine sample size second, how to determine the number of stimuli required for sufficient power and finally, how to conduct sample size planning without available data. In this tutorial, we discuss how to estimate power for mixed-effects models in different use cases: first, how to use models that were fit on available (e.g. Although various tools for conducting simulation-based power analyses for mixed-effects models are available, there is lack of guidance on how to appropriately use them. A flexible and very intuitive alternative to analytic power solutions are simulation-based power analyses. Being able to estimate this probability, however, is critical for sample size planning, as power is closely linked to the reliability and replicability of empirical findings. Mixed-effects models are a powerful tool for modeling fixed and random effects simultaneously, but do not offer a feasible analytic solution for estimating the probability that a test correctly rejects the null hypothesis.
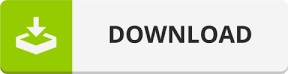